- Eric D. Brown, D.Sc.
- Posts
- AI Usage Patterns: What The Data Really Shows
AI Usage Patterns: What The Data Really Shows
AI adoption isn’t about wholesale job replacement. It’s about targeted augmentation. This study reveals where AI is actually making an impact and shows business leaders how to deploy it strategically for maximum value.
A recent study from Anthropic analyzed over 4 million Claude conversations to understand how AI is actually being used across different economic tasks and occupations. The findings offer valuable insights for business leaders looking to implement AI strategically.
Let's look at how AI tasks map to different occupational categories:

Figure 1: Analysis framework showing how AI conversations map to specific tasks and broader occupational categories. The diagram illustrates both direct task mapping and key analytical dimensions including wage correlation, automation patterns, and skill requirements.
This mapping reveals patterns in how AI is being deployed across different sectors. The study shows clear pathways from user interactions to specific occupational tasks and broader categories. For example, when a user asks for help optimizing game settings, this maps to computer and mathematical tasks. Similarly, content review requests align with arts and media functions.
The granular breakdown by occupational category provides even more insight:
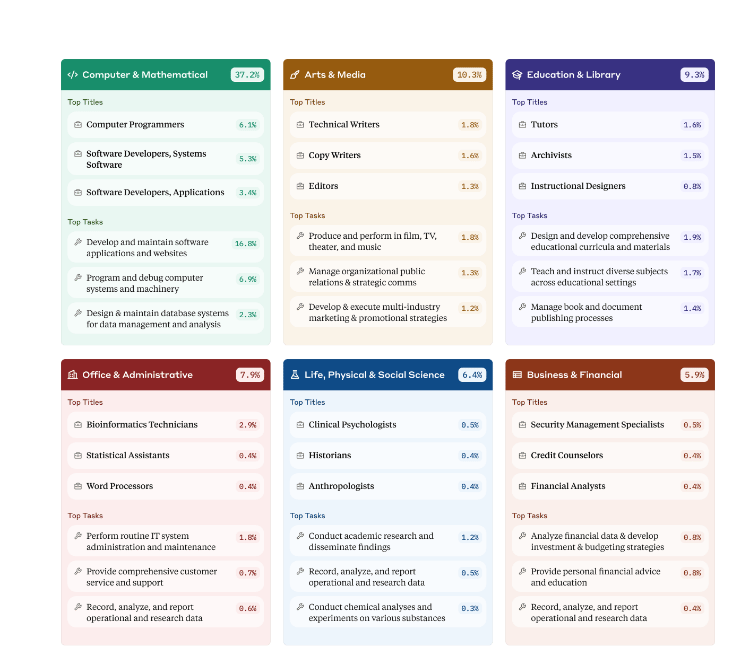
Figure 2: Detailed analysis of AI usage across major occupational categories, showing the percentage of total AI usage, top job titles, and most common tasks within each category. Computer & Mathematical occupations show the highest usage at 37.2%, with software development and programming tasks dominating the activity.
Computer & Mathematical occupations dominate AI usage at 37.2%, with roles like computer programmers (6.3%) and software developers (5.2%) leading the way. The most common tasks involve developing and maintaining software applications (16.1%) and programming/debugging computer systems (6.9%).
Arts & Media follows at 10.3%, where technical writers (1.8%) and copy writers (1.6%) frequently engage with AI for content creation and review. Education & Library occupations show similar usage levels at 9.2%, with tutors and archivists leveraging AI for educational content development and document management.
While many organizations are experimenting with AI, deep integration remains rare. Only about 4% of occupations show AI usage across 75% or more of their tasks. However, broader but shallower adoption is more common, with 36% of occupations using AI for at least a quarter of their tasks.
The highest AI usage appears in mid-to-high wage occupations requiring substantial but not elite-level specialization. Both very high-wage positions (like physicians) and low-wage roles show notably lower AI usage rates, likely due to regulatory constraints and the physical nature of many tasks respectively.
Perhaps most intriguingly, the study found that AI serves more as a collaborative tool than a replacement technology. About 57% of interactions showed patterns of augmentation (humans and AI working together iteratively) versus 43% showing automation (AI handling tasks independently). This pattern holds true across most occupational categories, suggesting that AI is primarily enhancing rather than replacing human capabilities.
The study also reveals interesting patterns in skill usage. Critical thinking and writing skills dominate AI interactions, while physical skills like installation and equipment maintenance show minimal presence. This aligns with AI's current strengths in information processing and content generation.
What does this mean for you?
For business leaders, these findings point toward focusing AI implementation on specific high-value tasks rather than attempting wholesale automation of roles. The data suggests starting with areas like software development, content creation, and analytical work where AI has proven most effective as a collaborative tool.
The key is understanding that current AI adoption follows a pattern of selective integration rather than comprehensive transformation. Organizations seeing the most success appear to be those using AI to augment human capabilities in specific domains rather than pursuing broader but shallower implementation.
As AI capabilities continue advancing, these usage patterns will likely evolve. Keeping track of where and how AI is actually being used effectively, rather than where it theoretically could be used, offers valuable guidance for strategic implementation decisions.
The research shows that AI adoption is currently targeted, not transformative. Success comes from identifying specific opportunities where AI enhances human capabilities, particularly in technical, creative, and analytical roles.
The full research paper provides additional details and methodological information. The authors note important limitations in their dataset and analysis methods, but the broad patterns identified align with what many organizations are experiencing in practice.
For now, the roadmap for leaders is clear. Focus on strategic integration in areas where AI adds real value rather than chasing wholesale automation. The data shows this targeted approach works.
What do you think? How does this report’s findings align with AI usage patterns you're seeing in your organization?
Let me know in the comments below.
If you found this post helpful, consider sharing it with another executive grappling with AI, technology, and data. If you want to explore AI and other Technology strategies, grab some time on my calendar, and let's chat.
Your next read
The constant flood of AI announcements and breakthroughs can feel overwhelming, even for experienced technology leaders. Here's a practical approach to staying current without getting lost in the hype.
Reply